A data warehouse is typically made up of fact and dimension tables that are loaded using different load patterns such as incremental vs. full; and batched vs real-time. The different types of fact and dimension tables can further influence how the data warehouse gets loaded. Slowly Changing Dimensions (SCD) is one type of dimension table found in a data warehouse. It simply works by storing, tracking and managing current and historical data over a period of time within a data warehouse.
In this article, we look at one example of a data warehouse load, specifically an incremental load of a type 2 Slowly Changing Dimension using a SQL Merge statement. SQL Merge is an ANSI standard (SQL:2003) supported by database systems such as Oracle, PostgreSQL and SQL Server. To demonstrate a load of Slowly Changing Dimension using a SQL Merge, we will use the following:
- SQL Server – specifically T-SQL within a Microsoft’s Azure SQL DB
- Premier League dataset
Premier League Use Case
The Premier League (also known as EPL) is England’s top football league system and one of the most watched football league around the globe. It is organized by seasons which run between August until May of the following year. A total of 38 games are played by each of the 20 teams participating in a season with the Premier League trophy awarded to a team that accumulated the highest points.
Historical and current season data is available for download from various sports websites. One such sports website is Eurosport.com which keeps track of league standings per season per team along with goals scored, games won, draws, losses, goals conceded etc. An example of Premier League standings data from Eurosport.com is shown in Figure 1.
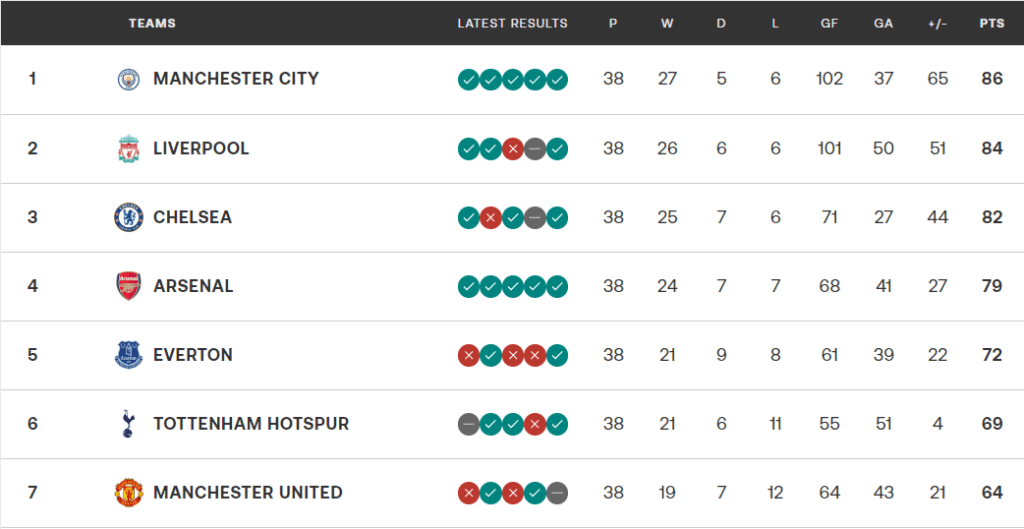
The Premier League use case is a perfect candidate to simulate data warehouse load as it has various data sets that could be used to apply different data warehouse load patterns. Teams in the Premier League refer to the different football clubs that play in the Premier League. Teams is therefore one data set that we could store in a dimension to simulate a data warehouse load. Possible attributes of a Team dimension are:
- Name of team
- Team description
- The season that the team plays in (although season can also be a standalone dimension).
Stage Data into Azure SQL DB
To get started, we extract the Premier League data shown in Figure 1 into a table in Azure SQL DB. The definition of our staging table is shown in Script 1.
CREATE TABLE [dbo].[PL_Season_2010_2011](
[NO] [tinyint] NOT NULL,
[TEAMS] [nvarchar](50) NOT NULL,
[P] [tinyint] NOT NULL,
[W] [tinyint] NOT NULL,
[D] [tinyint] NOT NULL,
[L] [tinyint] NOT NULL,
[GF] [tinyint] NOT NULL,
[GA] [tinyint] NOT NULL,
[GD] [smallint] NOT NULL,
[PTS] [tinyint] NOT NULL
) ON [PRIMARY]
GO
Script 1: CREATE TABLE Statement for the staging table
The simplest way to get the data staged would be to copy the data from Eurosport.com into Excel Spreadsheet and then import it using SSMS as shown in Figure 2.
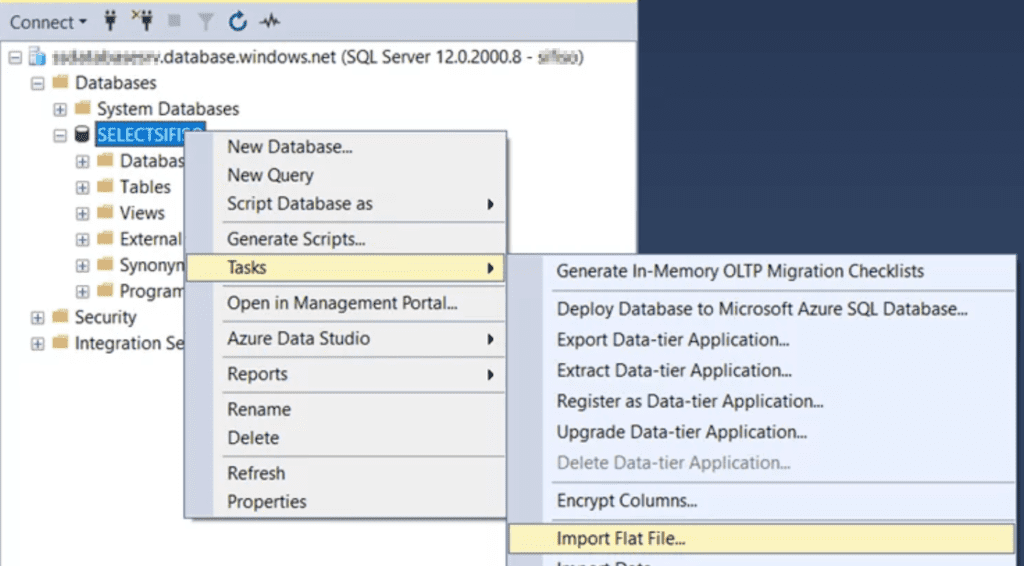
Figure 3 shows Premier League 2020-2011 season data that has been imported and staged into SQL table dbo.PL_Season_2010_2011.
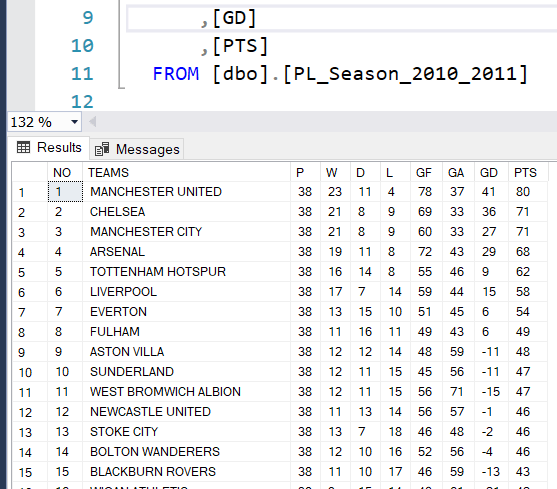
The rest of the different Premier League seasons’ data was successfully staged into separate tables as shown in Figure 4.
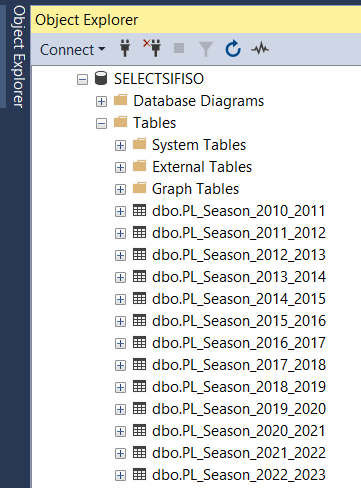
Let’s chat
Tell us about your idea, and we’ll offer the most fitting data solution.
Create Dimension Table
Data staging helps prepare the definition of the dimension table that will be used to store the final data. Script 2 provides a CREATE TABLE statement for our Team dimension. You will notice in Line 1 under Script 2 that the Team dimension is created under the DIM schema this is to make it easier to identify data warehouse dimension tables.
CREATE TABLE [DIM].[Team](
[TeamID] [int] IDENTITY(1,1) NOT NULL,
[Team] [varchar](255) NOT NULL,
[Season] [varchar](25) NOT NULL,
[IsActiveRecord] [bit] NULL,
[IsDeletedRecord] [bit] NULL DEFAULT ((0)) ,
[ActiveRecord_Start] [datetime2](7) NOT NULL DEFAULT (GETDATE()),
[ActiveRecord_End] [datetime2](7) NOT NULL DEFAULT ('2099-12-31')
) ON [PRIMARY]
GO
Script 2: CREATE TABLE Statement for Team dimension
The definition of our Team dimension consists of business-related fields such as Team (for team name) and Season. However, data warehouse designs typically recommend creation of metadata columns in addition to business key columns. Thus lines 2, 5-8 represent such metadata columns in our dimension. The complete name and description of the columns used in Script 2 are provided in Table 1.
Column Name | Column Description |
TeamID | is a surrogate key used to uniquely identify a record in the dimension |
Team | is used to store names of the Teams participating in Premier League Seasons. The team is also the unique business key identifier |
Season | is used to store the Premier League Seasons i.e. 2010-2011, 2011-2012 |
IsActiveRecord | is a Boolean value used to indicate current and historical values. Current values are indicated by one (1) whereas a zero (0) indicates historical record. |
IsDeletedRecord | is used to indicate a record that no longer exists at source. |
ActiveRecord_Start | is used to track the effective start date for when the record is current/active. All records are assigned a default value of the current date until they are closed off and replaced by the latest versions of the records |
ActiveRecord_End | is used to track effective end dates for when the record is current/active. If the record remains active then the default value is set to a future date of 2099-12-31 |
Build SQL Merge Statement
Now that we have prepared our staging dataset and created our Team dimension, we move next to build a SQL Merge statement that will be used to load the Team dimension. Generally, SQL Merge statements work by comparing source data (incoming records) against target data (existing records in a dimension table). If data at the source doesn’t exist at the target, then you can choose to insert the new data into the dimension.
Script 3 is a statement for preparing our source data using one of the Premier League season’s data that has previously been staged. The script simply returns a list of unique Teams from the 2010-2011 season’s data.
SELECT DISTINCT
[TEAMS] as [Team]
,'2010-2011' as Season
, GETDATE() AS ActiveRecord_Start
FROM [dbo].[PL_Season_2010_2011]
Script 3: CREATE TABLE Statement for Team dimension
The complete SQL Merge statement for our Team dimension is shown in Script 4. Some highlights of the SQL Merge statement are as follows:
- The join between source and target is based on the Team name. This comparison is only done against records that are currently active in the dimension.
- for WHEN MATCHED we run an update of the target provided that the team or season is not the same.
- The load into the data warehouse is from lines 29-46.
MERGE [DIM].[Team] AS TARGET
USING (
SELECT DISTINCT
[TEAMS] as [Team]
,'2010-2011' as Season
, GETDATE() AS ActiveRecord_Start
FROM [dbo].[PL_Season_2010_2011]
) AS SOURCE
ON (
TARGET.[Team] = SOURCE.[Team]
AND TARGET.IsActiveRecord = 1
)
WHEN MATCHED
AND (
TARGET.[Team] <> SOURCE.[Team]
OR TARGET.[Season] <> SOURCE.[Season]
)
THEN
UPDATE SET
TARGET.[Season] = SOURCE.[Season]
,TARGET.ActiveRecord_Start = SOURCE.ActiveRecord_Start
WHEN NOT MATCHED BY SOURCE
AND TARGET.IsActiveRecord = 1
THEN DELETE
WHEN NOT MATCHED BY TARGET THEN
--Add new records into the dimension
INSERT (
[Team]
,[Season]
,[IsActiveRecord]
,[IsDeletedRecord]
,[ActiveRecord_Start]
,[ActiveRecord_End]
)
VALUES(
SOURCE.[Team]
,SOURCE.[Season]
,1
,0
,SOURCE.ActiveRecord_Start
,'2099-12-31 23:59'
)
;
Script 4: CREATE TABLE Statement for Team dimension
Figure 5 shows the data loaded into the Team dimension following the execution of Script 4 – by default all the new records will have an IsActiveRecord flag of one (1), IsDeletedRecord to zero (0), and ActiveRecord_Start set to the current date.
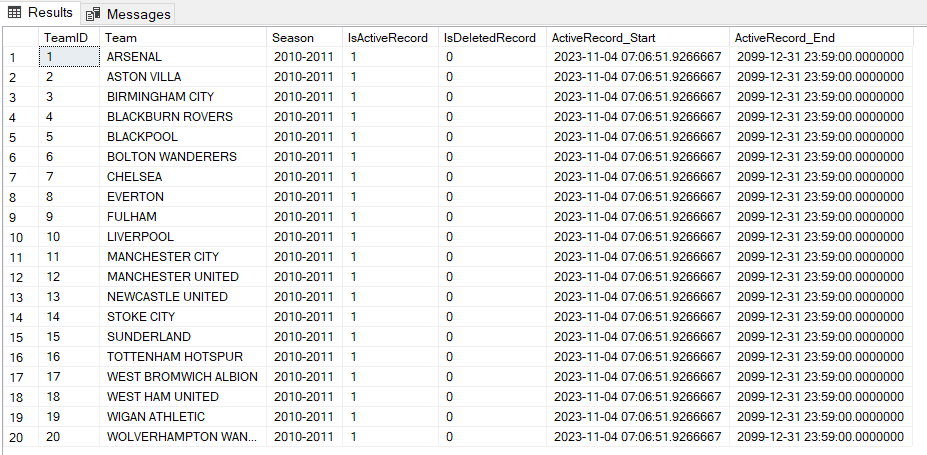
Implement Slowly Changing Dimension
At this point, we have successfully demonstrated the following:
- Prepare and Stage data into the data warehouse (hosted in SQL Azure DB)
- Create a target Team dimension under a seperate schema (DIM)
- Build source query to identify Premier League team records
- Build a SQL Merge statement to load Team dimension
What would happen if we were to load next season’s data from our staging like the 2011-12 season?
Well, the SQL Merge statement will do the following:
- Update the existing records
- Insert new Teams
- Delete teams no longer exist at the source
However, this will not version control our existing records – it would simply implement a type 1 SCD, which is replacing old records with new ones. As mentioned earlier, we aim to implement a type 2 SCD – which means we need to find a mechanism to close out existing records and flag new versions of the records as active.
The mechanism to do this in the SQL Merge is via the OUTPUT clause. OUTPUT variables are used to track the version of records that have been deleted, updated or inserted. We can extend our current type 1 SCD functionality by tracking all records that are deleted or updated by storing them in a temporary table and then writing those records back into the Team dimension.
Script 5 shows the complete SQL Merge stored procedure that implements type 2 SCD.
- Line 3 declares a temporary table variable that will be used to store records tracked by the OUTPUT clause.
- Lines 61-70 are used to write any updated/deleted/inserted records into the temporary table variable
- Lines 73-110 are then used to write the updated and deleted records back into our Team dimension.
CREATE PROC [DIM].[prcTeam] as
DECLARE @DIM_Team TABLE
(
ActionType [varchar](25) NULL,
[Team] [varchar](255) NULL,
[Season] [varchar](25) NULL,
[IsActiveRecord] [bit] NULL,
[IsDeletedRecord] [bit] NULL DEFAULT (0),
[ActiveRecord_Start] [datetime2](7) NULL,
[ActiveRecord_End] [datetime2](7) NULL
);
MERGE [DIM].[Team] AS TARGET
USING (
SELECT DISTINCT
[TEAMS] as [Team]
,'2011-2012' as Season
, GETDATE() AS ActiveRecord_Start
FROM [dbo].[PL_Season_2011_2012]
) AS SOURCE
ON (
TARGET.[Team] = SOURCE.[Team]
AND TARGET.IsActiveRecord = 1
)
WHEN MATCHED
AND (
TARGET.[Team] <> SOURCE.[Team]
OR TARGET.[Season] <> SOURCE.[Season]
)
THEN
UPDATE SET
TARGET.[Season] = SOURCE.[Season]
,TARGET.ActiveRecord_Start = SOURCE.ActiveRecord_Start
WHEN NOT MATCHED BY SOURCE
AND TARGET.IsActiveRecord = 1
THEN DELETE
WHEN NOT MATCHED BY TARGET THEN
--Add new records into the dimension
INSERT (
[Team]
,[Season]
,[IsActiveRecord]
,[IsDeletedRecord]
,[ActiveRecord_Start]
,[ActiveRecord_End]
)
VALUES(
SOURCE.[Team]
,SOURCE.[Season]
,1
,0
,SOURCE.ActiveRecord_Start
,'2099-12-31 23:59'
)
OUTPUT
$action AS ActionType
,DELETED.[Team]
,DELETED.[Season]
,DELETED.IsActiveRecord
,DELETED.[IsDeletedRecord]
,DELETED.[ActiveRecord_Start]
,GETDATE()
INTO @DIM_Team;
----expire previously active record
INSERT INTO [DIM].[Team]
(
[Team]
,[Season]
,[IsActiveRecord]
,IsDeletedRecord
,[ActiveRecord_Start]
,[ActiveRecord_End]
)
SELECT
[Team]
,[Season]
,0 as IsActiveRecord
,0 IsDeletedRecord
,[ActiveRecord_Start]
,[ActiveRecord_End]
FROM @DIM_Team
WHERE ActionType in ('UPDATE')
----expire previously active record
INSERT INTO [DIM].[Team]
(
[Team]
,[Season]
,[IsActiveRecord]
,IsDeletedRecord
,[ActiveRecord_Start]
,[ActiveRecord_End]
)
SELECT
[Team]
,[Season]
,0 as IsActiveRecord
,1 IsDeletedRecord
,[ActiveRecord_Start]
,[ActiveRecord_End]
FROM @DIM_Team
WHERE ActionType in ('DELETE')
;
GO
Script 5: SQL Merge Proc with OUTPUT Clause
Figure 6 shows a preview of our type 2 SCD Team dimension with 2-seasons’ worth of data (2010-2011 & 2011-2012 season data). You will notice for instance that the Arsenal team appears in both seasons as a result the latest season data (2011-2012) has an active record flag. Birmingham City team only appeared in the 2010-2011 season – as a result, it was then flagged as deleted during the processing of the 2011-2012 season data; the same is true of the Blackpool team at line 8 – these teams were relegated at the end of 2010-2011 season.
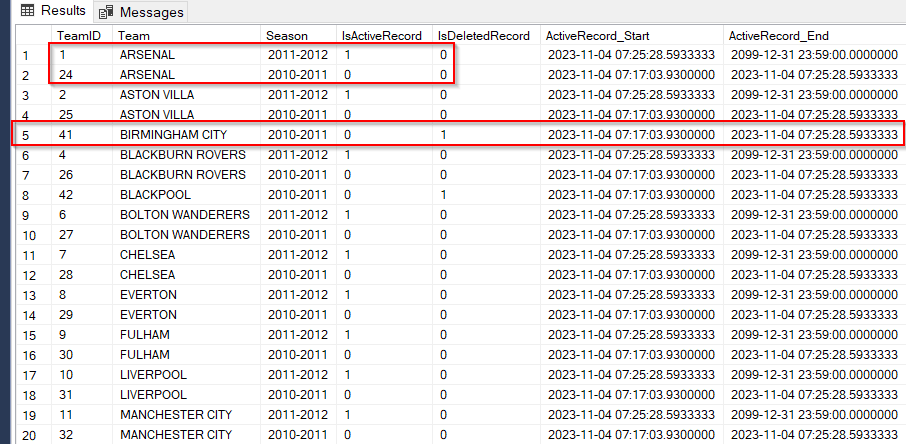
Summary
This article has demonstrated a data warehouse load using a SQL Merge statement. We prepared and staged the Premier League season’s data from the Eurosport.com website into a SQL Azure DB. We then created a target Team dimension and populated it using the SQL Merge statement.
Start your project
We help business build an intelligently designed digital future. Book a free 45 minutes consultation.
0 Comments